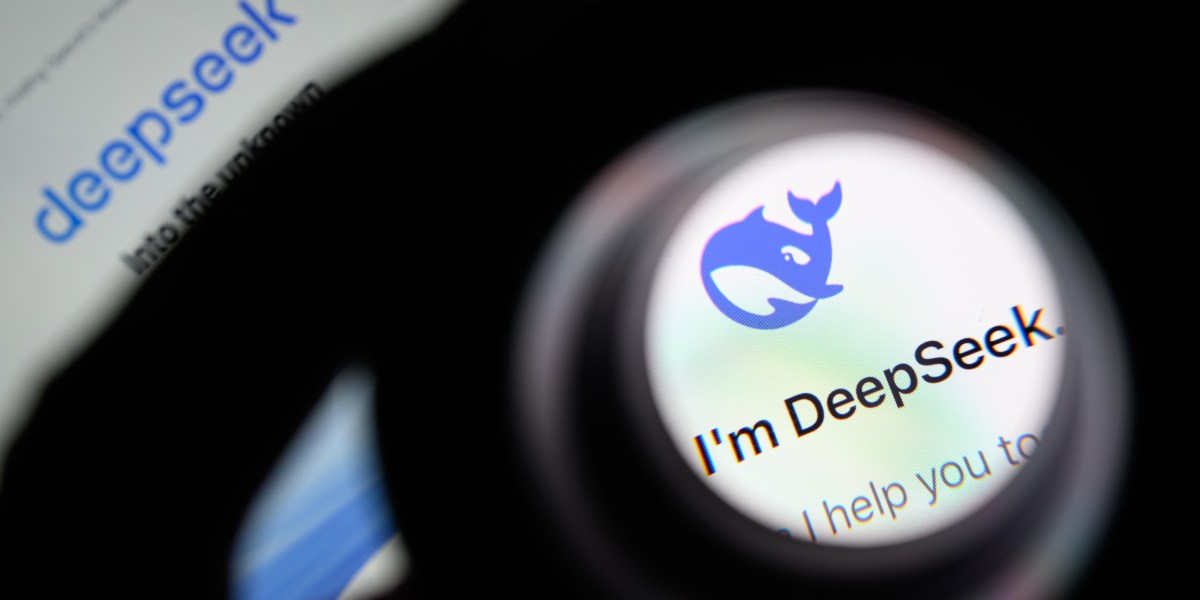
Within AI, though, what impact is DeepSeek likely to have in the longer term? Here are three seeds DeepSeek has planted that will grow even as the initial hype fades.
First, it’s forcing a debate about how much energy AI models should be allowed to use up in pursuit of better answers.
You may have heard (including from me) that DeepSeek is energy efficient. That’s true for its training phase, but for inference, which is when you actually ask the model something and it produces an answer, it’s complicated. It uses a chain-of-thought technique, which breaks down complex questions–-like whether it’s ever okay to lie to protect someone’s feelings—into chunks, and then logically answers each one. The method allows models like DeepSeek to do better at math, logic, coding, and more.
The problem, at least to some, is that this way of “thinking” uses up a lot more electricity than the AI we’ve been used to. Though AI is responsible for a small slice of total global emissions right now, there is increasing political support to radically increase the amount of energy going toward AI. Whether or not the energy intensity of chain-of-thought models is worth it, of course, depends on what we’re using the AI for. Scientific research to cure the world’s worst diseases seems worthy. Generating AI slop? Less so.
Some experts worry that the impressiveness of DeepSeek will lead companies to incorporate it into lots of apps and devices, and that users will ping it for scenarios that don’t call for it. (Asking DeepSeek to explain Einstein’s theory of relativity is a waste, for example, since it doesn’t require logical reasoning steps, and any typical AI chat model can do it with less time and energy.) Read more from me here.
Second, DeepSeek made some creative advancements in how it trains, and other companies are likely to follow its lead.
Advanced AI models don’t just learn on lots of text, images, and video. They rely heavily on humans to clean that data, annotate it, and help the AI pick better responses, often for paltry wages.
One way human workers are involved is through a technique called reinforcement learning with human feedback. The model generates an answer, human evaluators score that answer, and those scores are used to improve the model. OpenAI pioneered this technique, though it’s now used widely by the industry.
Recent Comments