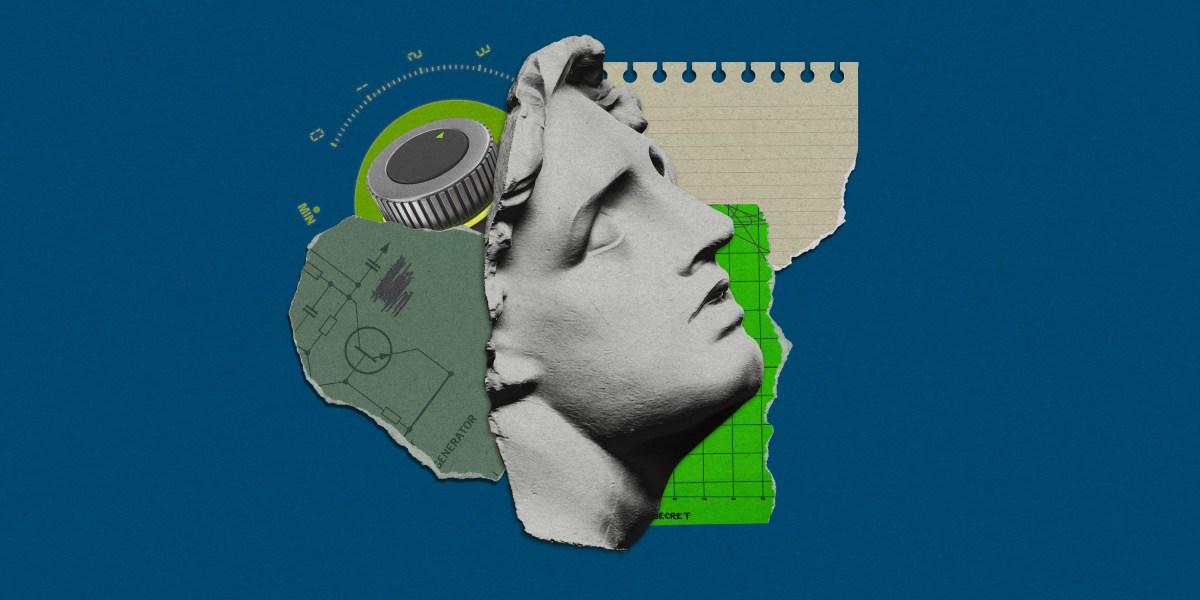
“We’ve been really pushing on ‘thinking,’” says Jack Rae, a principal research scientist at DeepMind. Such models, which are built to work through problems logically and spend more time arriving at an answer, rose to prominence earlier this year with the launch of the DeepSeek R1 model. They’re attractive to AI companies because they can make an existing model better by training it to approach a problem pragmatically. That way, the companies can avoid having to build a new model from scratch.
When the AI model dedicates more time (and energy) to a query, it costs more to run. Leaderboards of reasoning models show that one task can cost upwards of $200 to complete. The promise is that this extra time and money help reasoning models do better at handling challenging tasks, like analyzing code or gathering information from lots of documents.
“The more you can iterate over certain hypotheses and thoughts,” says Google DeepMind chief technical officer Koray Kavukcuoglu, the more “it’s going to find the right thing.”
This isn’t true in all cases, though. “The model overthinks,” says Tulsee Doshi, who leads the product team at Gemini, referring specifically to Gemini Flash 2.5, the model released today that includes a slider for developers to dial back how much it thinks. “For simple prompts, the model does think more than it needs to.”
When a model spends longer than necessary on a problem, it makes the model expensive to run for developers and worsens AI’s environmental footprint.
Nathan Habib, an engineer at Hugging Face who has studied the proliferation of such reasoning models, says overthinking is abundant. In the rush to show off smarter AI, companies are reaching for reasoning models like hammers even where there’s no nail in sight, Habib says. Indeed, when OpenAI announced a new model in February, it said it would be the company’s last nonreasoning model.
The performance gain is “undeniable” for certain tasks, Habib says, but not for many others where people normally use AI. Even when reasoning is used for the right problem, things can go awry. Habib showed me an example of a leading reasoning model that was asked to work through an organic chemistry problem. It started out okay, but halfway through its reasoning process the model’s responses started resembling a meltdown: It sputtered “Wait, but …” hundreds of times. It ended up taking far longer than a nonreasoning model would spend on one task. Kate Olszewska, who works on evaluating Gemini models at DeepMind, says Google’s models can also get stuck in loops.
Google’s new “reasoning” dial is one attempt to solve that problem. For now, it’s built not for the consumer version of Gemini but for developers who are making apps. Developers can set a budget for how much computing power the model should spend on a certain problem, the idea being to turn down the dial if the task shouldn’t involve much reasoning at all. Outputs from the model are about six times more expensive to generate when reasoning is turned on.
Recent Comments