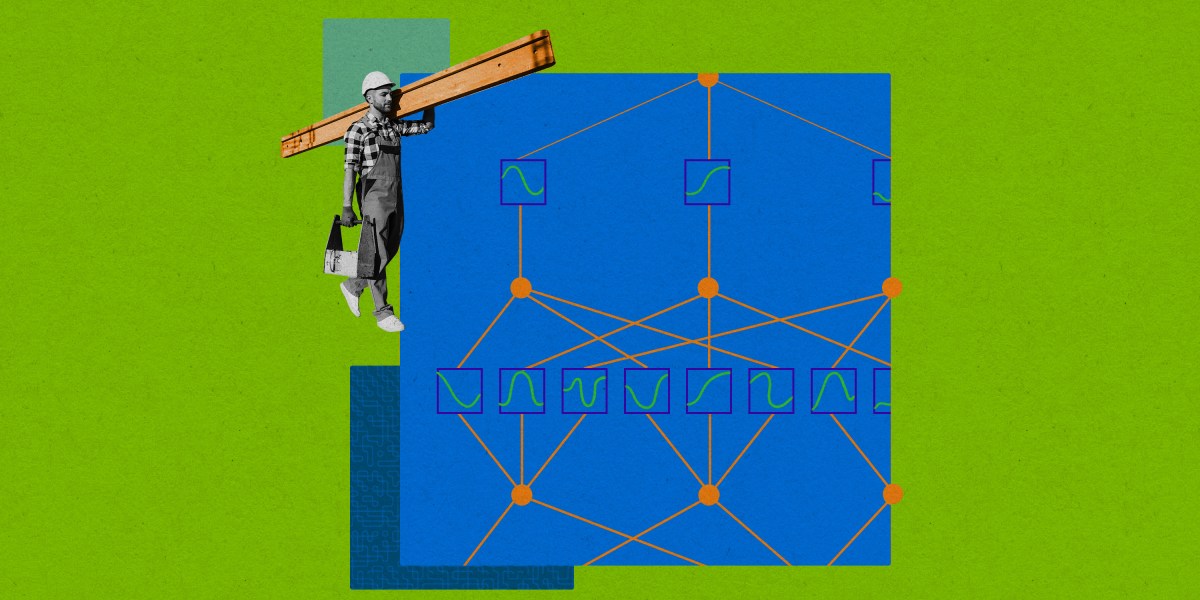
The simplification, studied in detail by a group led by researchers at MIT, could make it easier to understand why neural networks produce certain outputs, help verify their decisions, and even probe for bias. Preliminary evidence also suggests that as KANs are made bigger, their accuracy increases faster than networks built of traditional neurons.
“It’s interesting work,” says Andrew Wilson, who studies the foundations of machine learning at New York University. “It’s nice that people are trying to fundamentally rethink the design of these [networks].”
The basic elements of KANs were actually proposed in the 1990s, and researchers kept building simple versions of such networks. But the MIT-led team has taken the idea further, showing how to build and train bigger KANs, performing empirical tests on them, and analyzing some KANs to demonstrate how their problem-solving ability could be interpreted by humans. “We revitalized this idea,” said team member Ziming Liu, a PhD student in Max Tegmark’s lab at MIT. “And, hopefully, with the interpretability… we [may] no longer [have to] think neural networks are black boxes.”
While it’s still early days, the team’s work on KANs is attracting attention. GitHub pages have sprung up that show how to use KANs for myriad applications, such as image recognition and solving fluid dynamics problems.
Finding the formula
The current advance came when Liu and colleagues at MIT, Caltech, and other institutes were trying to understand the inner workings of standard artificial neural networks.
Today, almost all types of AI, including those used to build large language models and image recognition systems, include sub-networks known as a multilayer perceptron (MLP). In an MLP, artificial neurons are arranged in dense, interconnected “layers.” Each neuron has within it something called an “activation function”—a mathematical operation that takes in a bunch of inputs and transforms them in some pre-specified manner into an output.
Recent Comments