The potential of artificial intelligence (AI) and machine learning (ML) seems almost unbounded in its ability to derive and drive new sources of customer, product, service, operational, environmental, and societal value. If your organization is to compete in the economy of the future, then AI must be at the core of your business operations.
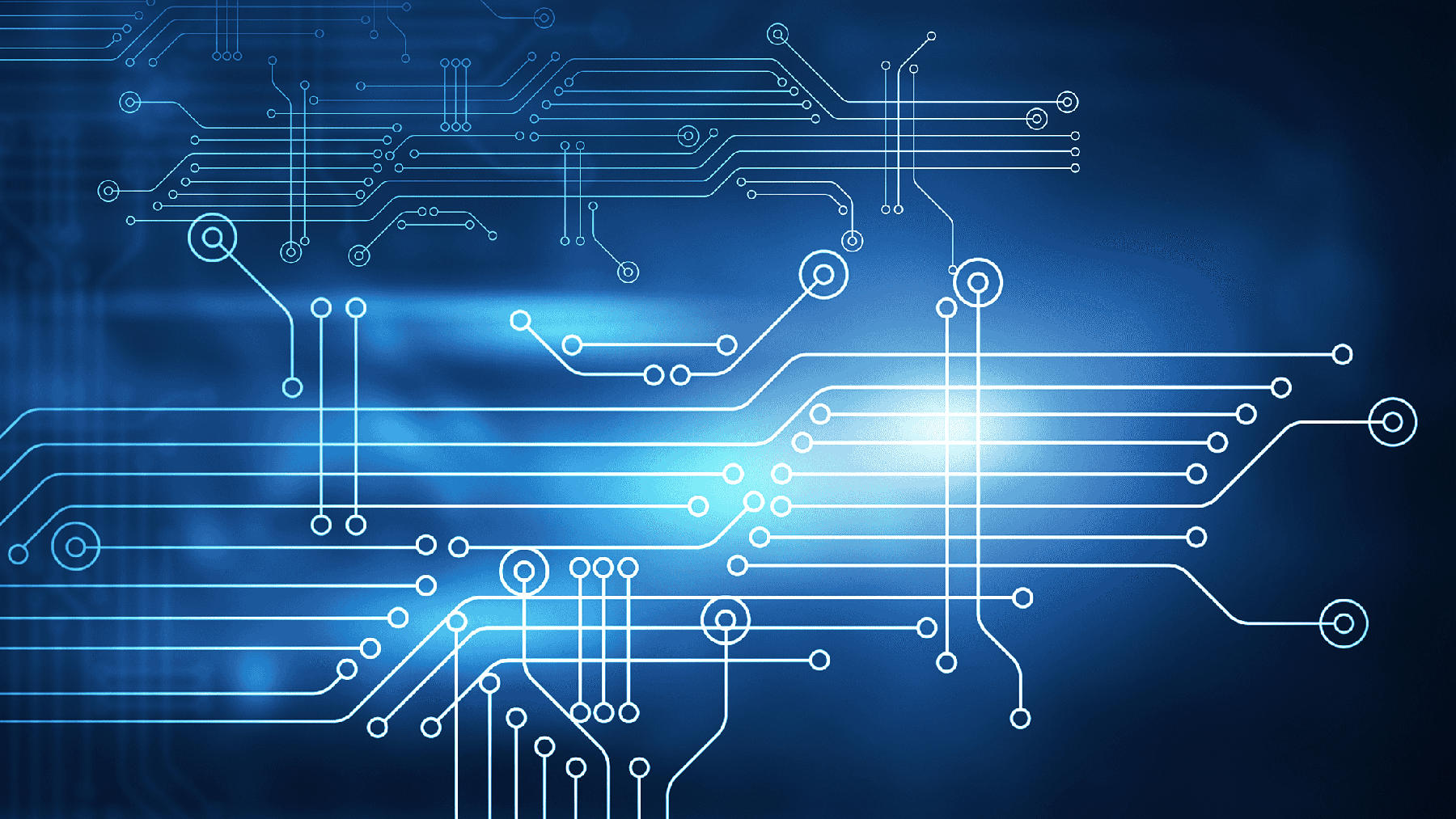
A study by Kearney titled “The Impact of Analytics in 2020” highlights the untapped profitability and business impact for organizations looking for justification to accelerate their data science (AI / ML) and data management investments:
- Explorers could improve profitability by 20% if they were as effective as Leaders
- Followers could improve profitability by 55% if they were as effective as Leaders
- Laggards could improve profitability by 81% if they were as effective as Leaders
The business, operational, and societal impacts could be staggering except for one significant organizational challenge—data. No one less than the godfather of AI, Andrew Ng, has noted the impediment of data and data management in empowering organizations and society in realizing the potential of AI and ML:
“The model and the code for many applications are basically a solved problem. Now that the models have advanced to a certain point, we’ve got to make the data work as well.” — Andrew Ng
Data is the heart of training AI and ML models. And high-quality, trusted data orchestrated through highly efficient and scalable pipelines means that AI can enable these compelling business and operational outcomes. Just like a healthy heart needs oxygen and reliable blood flow, so too is a steady stream of cleansed, accurate, enriched, and trusted data important to the AI / ML engines.
For example, one CIO has a team of 500 data engineers managing over 15,000 extract, transform, and load (ETL) jobs that are responsible for acquiring, moving, aggregating, standardizing, and aligning data across 100s of special-purpose data repositories (data marts, data warehouses, data lakes, and data lakehouses). They’re performing these tasks in the organization’s operational and customer-facing systems under ridiculously tight service level agreements (SLAs) to support their growing number of diverse data consumers. It seems Rube Goldberg certainly must have become a data architect (Figure 1).
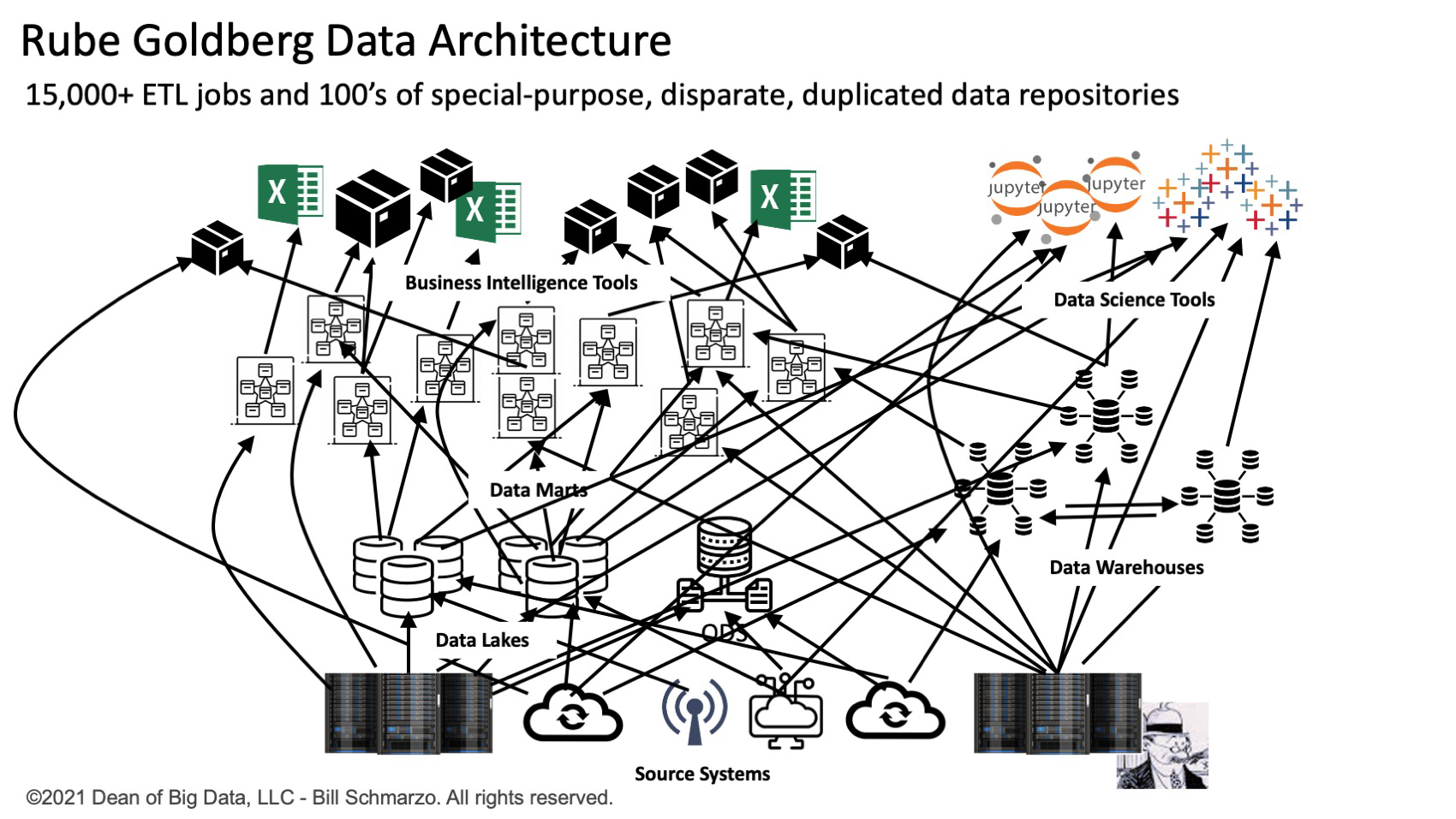
Reducing the debilitating spaghetti architecture structures of one-off, special-purpose, static ETL programs to move, cleanse, align, and transform data is greatly inhibiting the “time to insights” necessary for organizations to fully exploit the unique economic characteristics of data, the “world’s most valuable resource” according to The Economist.
Emergence of intelligent data pipelines
The purpose of a data pipeline is to automate and scale common and repetitive data acquisition, transformation, movement, and integration tasks. A properly constructed data pipeline strategy can accelerate and automate the processing associated with gathering, cleansing, transforming, enriching, and moving data to downstream systems and applications. As the volume, variety, and velocity of data continue to grow, the need for data pipelines that can linearly scale within cloud and hybrid cloud environments is becoming increasingly critical to the operations of a business.
A data pipeline refers to a set of data processing activities that integrates both operational and business logic to perform advanced sourcing, transformation, and loading of data. A data pipeline can run on either a scheduled basis, in real time (streaming), or be triggered by a predetermined rule or set of conditions.
Additionally, logic and algorithms can be built into a data pipeline to create an “intelligent” data pipeline. Intelligent pipelines are reusable and extensible economic assets that can be specialized for source systems and perform the data transformations necessary to support the unique data and analytic requirements for the target system or application.
As machine learning and AutoML become more prevalent, data pipelines will increasingly become more intelligent. Data pipelines can move data between advanced data enrichment and transformation modules, where neural network and machine learning algorithms can create more advanced data transformations and enrichments. This includes segmentation, regression analysis, clustering, and the creation of advanced indices and propensity scores.
Finally, one could integrate AI into the data pipelines such that they could continuously learn and adapt based upon the source systems, required data transformations and enrichments, and the evolving business and operational requirements of the target systems and applications.
For example: an intelligent data pipeline in health care could analyze the grouping of health care diagnosis-related groups (DRG) codes to ensure consistency and completeness of DRG submissions and detect fraud as the DRG data is being moved by the data pipeline from the source system to the analytic systems.
Realizing business value
Chief data officers and chief data analytic officers are being challenged to unleash the business value of their data—to apply data to the business to drive quantifiable financial impact.
The ability to get high-quality, trusted data to the right data consumer at the right time in order to facilitate more timely and accurate decisions will be a key differentiator for today’s data-rich companies. A Rube Goldberg system of ELT scripts and disparate, special analytic-centric repositories hinders an organizations’ ability to achieve that goal.
Learn more about intelligent data pipelines in Modern Enterprise Data Pipelines (eBook) by Dell Technologies here.
This content was produced by Dell Technologies. It was not written by MIT Technology Review’s editorial staff.
Recent Comments