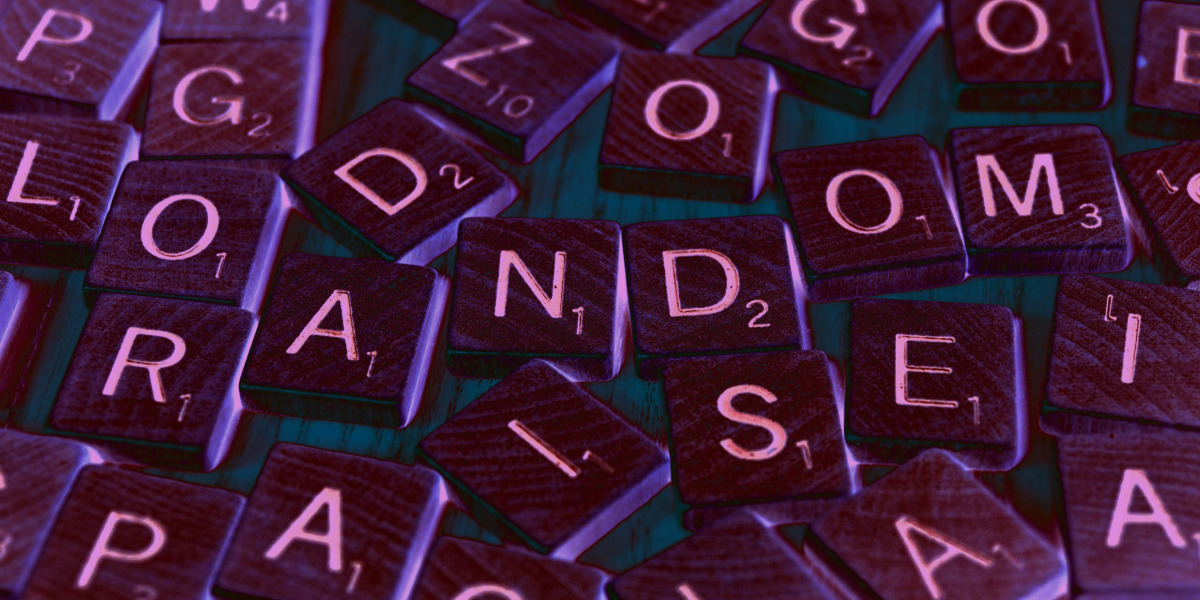
Researchers at Auburn University in Alabama and Adobe Research discovered the flaw when they tried to get an NLP system to generate explanations for its behavior, such as why it claimed different sentences meant the same thing. When they tested their approach, they realized that shuffling words in a sentence made no difference to the explanations. “This is a general problem to all NLP models,” says Anh Nguyen at Auburn University, who led the work.
The team looked at several state-of-the-art NLP systems based on BERT (a language model developed by Google that underpins many of the latest systems, including GPT-3). All of these systems score better than humans on GLUE (General Language Understanding Evaluation), a standard set of tasks designed to test language comprehension, such as spotting paraphrases, judging if a sentence expresses positive or negative sentiments, and verbal reasoning.
Man bites dog: They found that these systems couldn’t tell when words in a sentence were jumbled up, even when the new order changed the meaning. For example, the systems correctly spotted that the sentences “Does marijuana cause cancer?” and “How can smoking marijuana give you lung cancer?” were paraphrases. But they were even more certain that “You smoking cancer how marijuana lung can give?” and “Lung can give marijuana smoking how you cancer?” meant the same thing too. The systems also decided that sentences with opposite meanings—such as “Does marijuana cause cancer?” and “Does cancer cause marijuana?”—were asking the same question.
The only task where word order mattered was one in which the models had to check the grammatical structure of a sentence. Otherwise, between 75% and 90% of the tested systems’ answers did not change when the words were shuffled.
What’s going on? The models appear to pick up on a few key words in a sentence, whatever order they come in. They do not understand language as we do, and GLUE—a very popular benchmark—does not measure true language use. In many cases, the task a model is trained on does not force it to care about word order or syntax in general. In other words, GLUE teaches NLP models to jump through hoops.
Many researchers have started to use a harder set of tests called SuperGLUE, but Nguyen suspects it will have similar problems.
This issue has also been identified by Yoshua Bengio and colleagues, who found that reordering words in a conversation sometimes did not change the responses chatbots made. And a team from Facebook AI Research found examples of this happening with Chinese. Nguyen’s team shows that the problem is widespread.
Does it matter? It depends on the application. On one hand, an AI that still understands when you make a typo or say something garbled, as another human could, would be useful. But in general, word order is crucial when unpicking a sentence’s meaning.
fix it How to? The good news is that it might not be too hard to fix. The researchers found that forcing a model to focus on word order, by training it to do a task where word order mattered (such as spotting grammatical errors), also made the model perform better on other tasks. This suggests that tweaking the tasks that models are trained to do will make them better overall.
Nguyen’s results are yet another example of how models often fall far short of what people believe they’re capable of. He thinks it highlights how hard it is to make AIs that understand and reason like humans. “Nobody has a clue,” he says.
Recent Comments