But what computers were bad at, traditionally, was strategy—the ability to ponder the shape of a game many, many moves in the future. That’s where humans still had the edge.
Or so Kasparov thought, until Deep Blue’s move in game 2 rattled him. It seemed so sophisticated that Kasparov began worrying: maybe the machine was far better than he’d thought! Convinced he had no way to win, he resigned the second game.
But he shouldn’t have. Deep Blue, it turns out, wasn’t actually that good. Kasparov had failed to spot a move that would have let the game end in a draw. He was psyching himself out: worried that the machine might be far more powerful than it really was, he had begun to see human-like reasoning where none existed.
Knocked off his rhythm, Kasparov kept playing worse and worse. He psyched himself out over and over again. Early in the sixth, winner-takes-all game, he made a move so lousy that chess observers cried out in shock. “I was not in the mood of playing at all,” he later said at a press conference.
IBM benefited from its moonshot. In the press frenzy that followed Deep Blue’s success, the company’s market cap rose $11.4 billion in a single week. Even more significant, though, was that IBM’s triumph felt like a thaw in the long AI winter. If chess could be conquered, what was next? The public’s mind reeled.
“That,” Campbell tells me, “is what got people paying attention.”
The truth is, it wasn’t surprising that a computer beat Kasparov. Most people who’d been paying attention to AI—and to chess—expected it to happen eventually.
Chess may seem like the acme of human thought, but it’s not. Indeed, it’s a mental task that’s quite amenable to brute-force computation: the rules are clear, there’s no hidden information, and a computer doesn’t even need to keep track of what happened in previous moves. It just assesses the position of the pieces right now.
“There are very few problems out there where, as with chess, you have all the information you could possibly need to make the right decision.”
Everyone knew that once computers got fast enough, they’d overwhelm a human. It was just a question of when. By the mid-’90s, “the writing was already on the wall, in a sense,” says Demis Hassabis, head of the AI company DeepMind, part of Alphabet.
Deep Blue’s victory was the moment that showed just how limited hand-coded systems could be. IBM had spent years and millions of dollars developing a computer to play chess. But it couldn’t do anything else.
“It didn’t lead to the breakthroughs that allowed the [Deep Blue] AI to have a huge impact on the world,” Campbell says. They didn’t really discover any principles of intelligence, because the real world doesn’t resemble chess. “There are very few problems out there where, as with chess, you have all the information you could possibly need to make the right decision,” Campbell adds. “Most of the time there are unknowns. There’s randomness.”
But even as Deep Blue was mopping the floor with Kasparov, a handful of scrappy upstarts were tinkering with a radically more promising form of AI: the neural net.
With neural nets, the idea was not, as with expert systems, to patiently write rules for each decision an AI will make. Instead, training and reinforcement strengthen internal connections in rough emulation (as the theory goes) of how the human brain learns.
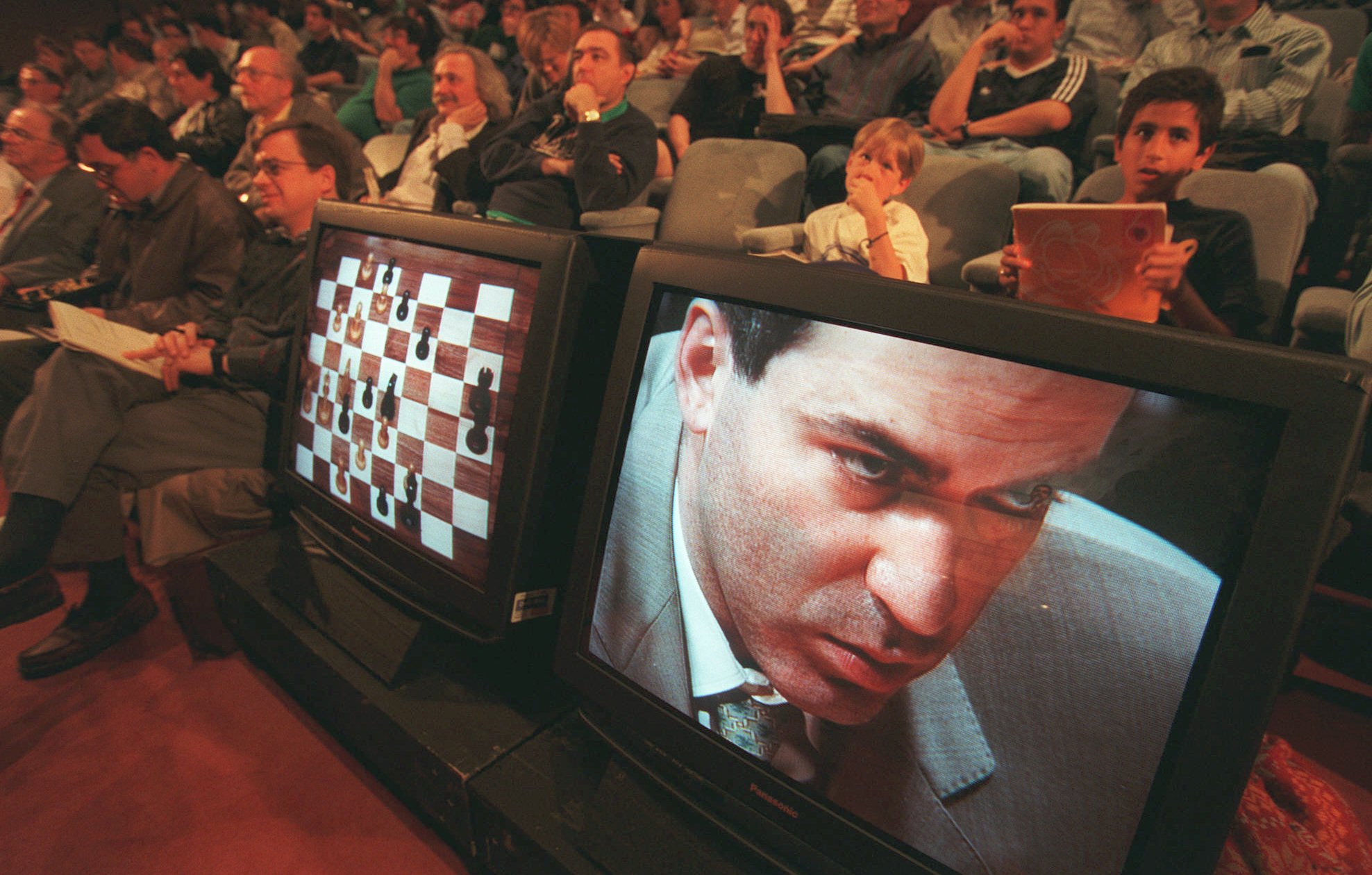
AP PHOTO / ADAM NADEL
The idea had existed since the ’50s. But training a usefully large neural net required lightning-fast computers, tons of memory, and lots of data. None of that was readily available then. Even into the ’90s, neural nets were considered a waste of time.
“Back then, most people in AI thought neural nets were just rubbish,” says Geoff Hinton, an emeritus computer science professor at the University of Toronto, and a pioneer in the field. “I was called a ‘true believer’”—not a compliment.
But by the 2000s, the computer industry was evolving to make neural nets viable. Video-game players’ lust for ever-better graphics created a huge industry in ultrafast graphic-processing units, which turned out to be perfectly suited for neural-net math. Meanwhile, the internet was exploding, producing a torrent of pictures and text that could be used to train the systems.
By the early 2010s, these technical leaps were allowing Hinton and his crew of true believers to take neural nets to new heights. They could now create networks with many layers of neurons (which is what the “deep” in “deep learning” means). In 2012 his team handily won the annual Imagenet competition, where AIs compete to recognize elements in pictures. It stunned the world of computer science: self-learning machines were finally viable.
Ten years into the deep-learning revolution, neural nets and their pattern-recognizing abilities have colonized every nook of daily life. They help Gmail autocomplete your sentences, help banks detect fraud, let photo apps automatically recognize faces, and—in the case of OpenAI’s GPT-3 and DeepMind’s Gopher—write long, human-sounding essays and summarize texts. They’re even changing how science is done; in 2020, DeepMind debuted AlphaFold2, an AI that can predict how proteins will fold—a superhuman skill that can help guide researchers to develop new drugs and treatments.
Meanwhile Deep Blue vanished, leaving no useful inventions in its wake. Chess playing, it turns out, wasn’t a computer skill that was needed in everyday life. “What Deep Blue in the end showed was the shortcomings of trying to handcraft everything,” says DeepMind founder Hassabis.
IBM tried to remedy the situation with Watson, another specialized system, this one designed to tackle a more practical problem: getting a machine to answer questions. It used statistical analysis of massive amounts of text to achieve language comprehension that was, for its time, cutting-edge. It was more than a simple if-then system. But Watson faced unlucky timing: it was eclipsed only a few years later by the revolution in deep learning, which brought in a generation of language-crunching models far more nuanced than Watson’s statistical techniques.
Deep learning has run roughshod over old-school AI precisely because “pattern recognition is incredibly powerful,” says Daphne Koller, a former Stanford professor who founded and runs Insitro, which uses neural nets and other forms of machine learning to investigate novel drug treatments. The flexibility of neural nets—the wide variety of ways pattern recognition can be used—is the reason there hasn’t yet been another AI winter. “Machine learning has actually delivered value,” she says, which is something the “previous waves of exuberance” in AI never did.
The inverted fortunes of Deep Blue and neural nets show how bad we were, for so long, at judging what’s hard—and what’s valuable—in AI.
For decades, people assumed mastering chess would be important because, well, chess is hard for humans to play at a high level. But chess turned out to be fairly easy for computers to master, because it’s so logical.
What was far harder for computers to learn was the casual, unconscious mental work that humans do—like conducting a lively conversation, piloting a car through traffic, or reading the emotional state of a friend. We do these things so effortlessly that we rarely realize how tricky they are, and how much fuzzy, grayscale judgment they require. Deep learning’s great utility has come from being able to capture small bits of this subtle, unheralded human intelligence.
Still, there’s no final victory in artificial intelligence. Deep learning may be riding high now—but it’s amassing sharp critiques, too.
“For a very long time, there was this techno-chauvinist enthusiasm that okay, AI is going to solve every problem!” says Meredith Broussard, a programmer turned journalism professor at New York University and author of Artificial Unintelligence. But as she and other critics have pointed out, deep-learning systems are often trained on biased data—and absorb those biases. The computer scientists Joy Buolamwini and Timnit Gebru discovered that three commercially available visual AI systems were terrible at analyzing the faces of darker-skinned women. Amazon trained an AI to vet résumés, only to find it downranked women.
Though computer scientists and many AI engineers are now aware of these bias problems, they’re not always sure how to deal with them. On top of that, neural nets are also “massive black boxes,” says Daniela Rus, a veteran of AI who currently runs MIT’s Computer Science and Artificial Intelligence Laboratory. Once a neural net is trained, its mechanics are not easily understood even by its creator. It is not clear how it comes to its conclusions—or how it will fail.
“For a very long time, there was this techno-chauvinist enthusiasm that Okay, AI is going to solve every problem!”
It may not be a problem, Rus figures, to rely on a black box for a task that isn’t “safety critical.” But what about a higher-stakes job, like autonomous driving? “It’s actually quite remarkable that we could put so much trust and faith in them,” she says.
This is where Deep Blue had an advantage. The old-school style of handcrafted rules may have been brittle, but it was comprehensible. The machine was complex—but it wasn’t a mystery.
Ironically, that old style of programming might stage something of a comeback as engineers and computer scientists grapple with the limits of pattern matching.
Language generators, like OpenAI’s GPT-3 or DeepMind’s Gopher, can take a few sentences you’ve written and keep on going, writing pages and pages of plausible-sounding prose. But despite some impressive mimicry, Gopher “still doesn’t really understand what it’s saying,” Hassabis says. “Not in a true sense.”
Similarly, visual AI can make terrible mistakes when it encounters an edge case. Self-driving cars have slammed into fire trucks parked on highways, because in all the millions of hours of video they’d been trained on, they’d never encountered that situation. Neural nets have, in their own way, a version of the “brittleness” problem.
Recent Comments